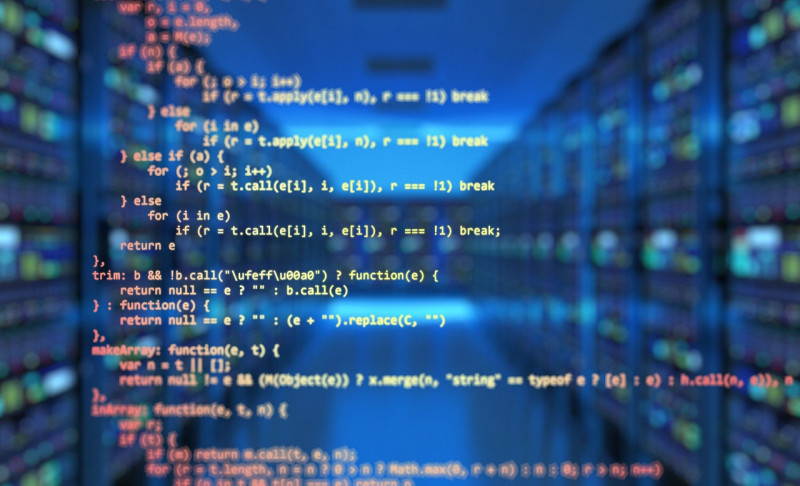
A gentle introduction to Bayesian Estimation
This course introduces all the essential ingredients needed to start Bayesian estimation and inference.
This course introduces all the essential ingredients needed to start Bayesian estimation and inference.
almost fully booked
We discuss specifying priors, obtaining the posterior, prior/posterior predictive checking, sensitivity analyses, and the usefulness of a specific class of priors called shrinkage priors. We propose strategies for reproducibility and reporting standards, outlining the WAMBS-checklist (when to Worry and how to Avoid the Misuse of Bayesian Statistics). We have prepared many exercises to enable students to get hands-on experience.
The popularity of Bayesian statistics has increased over the years. However, Bayesian methods are not a part of the statistics curricula in most graduate programmes internationally. The Bayesian framework can handle some commonly encountered problems in classical statistics, such as the lack of power in small sample research and convergence issues in complex models. Furthermore, some researchers prefer the Bayesian framework because it sequentially updates knowledge with new data instead of requiring that each new study tests the null hypothesis that there is no effect in the population. The main focus of the course is on conceptually understanding Bayesian inference and applying Bayesian methods.
The instructors will clarify the differences between the philosophies and interpretations in classical and Bayesian frameworks. They illustrate how different types of research questions can be answered using Bayesian methods. This course will also give students experience running Bayesian analyses and interpreting results and instruct participants on the prevailing 'best practices' for writing a scientific article based on Bayesian statistics. Participants will emerge from the course with knowledge about how to apply Bayesian methods to answer their research questions and with the ability to understand articles that examine and use Bayesian methods.
Day 1: we discuss the stages involved in Bayesian analysis: obtaining background knowledge from previous literature, specifying the prior distributions, and deriving the posterior. We discuss the importance of prior and posterior predictive checking, and selecting a proper technique for sampling from a probability distribution. We prepared exercises in web apps to play around with priors and data to learn how these will affect the posterior.
Day 2: we discuss reproducibility and reporting standards strategies, outlining the WAMBS-checklist (when to Worry and how to Avoid the Misuse of Bayesian Statistics). We have prepared exercises in R to get hands-on experience. If you are not familiar with R, we will send you some exercises to be completed before starting the course.
Day 3: we discuss estimation methods including alternatives that can be more efficient when dealing with computational or non-convergence issues. A brief introduction to and the benefits of these estimation methods (MCMC, Gibbs, MH, HMC, NUTS, etc.) will be reviewed. These insights can help to understand differences between software that can be used. Today may be a little more technical (less gentle) but without equations.
Day 4: although the prior distribution can offer many advantages, the prior can also inadvertently influence the results. Today we will discuss the importance of prior sensitivity analysis to investigate the influence the prior has on the results. We will focus on models with many parameters to estimate, possibly too many for the model to be identified in a classical sense. We discuss the use of shrinkage priors to estimate these models and select relevant parameters.
Day 5: today we elaborate on ways in which informative priors can be specified. We discuss how we can use expert knowledge and previous studies to inform these decisions. We also provide case studies and end with general reflections.
Among our Methodology and Statistics postgraduate courses, there is another course that covers Bayes: Beyond null-hypothesis testing: Transparent and informative methods in three statistical frameworks
This course focuses on hypothesis testing (instead of prediction) which also contains Bayesian hypothesis testing, using Bayes factors and posterior model probabilities.
The course takes place in the week after our course and may be a nice sequel.
Sara van Erp and Duco Veen
Knowledge of regression analysis is required. No previous knowledge of Bayesian analysis is assumed.
You do not need to know matrix algebra, calculus, or likelihood theory. The main focus is on conceptually understanding Bayesian statistics and applying Bayesian methods in R. If you are not familiar with R, we will send you some exercises to be completed before starting the course.
Participants from various fields—including psychology, education, human development, public health, prevention science, sociology, marketing, business, biology, medicine, political science, and communication—will benefit from the course.
For IOPS students who are taking this course there is an additional assignment that consists of analysing your own data using Bayesian analysis and reporting the results according to the reporting guidelines we discuss.
For an overview of all our summer school courses offered by the Department of Methodology and Statistics please click here.
We also offer tailor-made M&S courses and in-house M&S training. If you want to check out the possibilities, please contact us at ms.summerschool@uu.nl
After engaging in course lectures and discussions as well as completing the hands-on practice activities with empirical data, participants will be able to:
Participants will also complete the course with a foundation for future learning about Bayesian modeling and knowledge about available resources to guide such endeavours.
Please note that there are no graded activities included in this course. Therefore, we are not able to provide students with a transcript of grades. You will obtain a certificate upon completion of this course.
PhD students from the Faculty of Social and Behavioural Sciences at Utrecht University have the opportunity to attend three Winter/Summer School courses funded by the Graduate School of Social and Behavioural Sciences. Additionally, they may choose to take as many courses as they wish at their own expense from their personal budget..
There are no scholarships available for this course.
Housing for August 12 to August 23 is fully booked for new applications. For hotel and hostel options click here.
The housing costs do not include a Utrecht Summer School sleeping bag. This is a separate product on the invoice. If you wish to bring your own bedding, please deselect or remove the sleeping bag from your order.
If you are an IOPS or SMiP member, please mention this in your application.