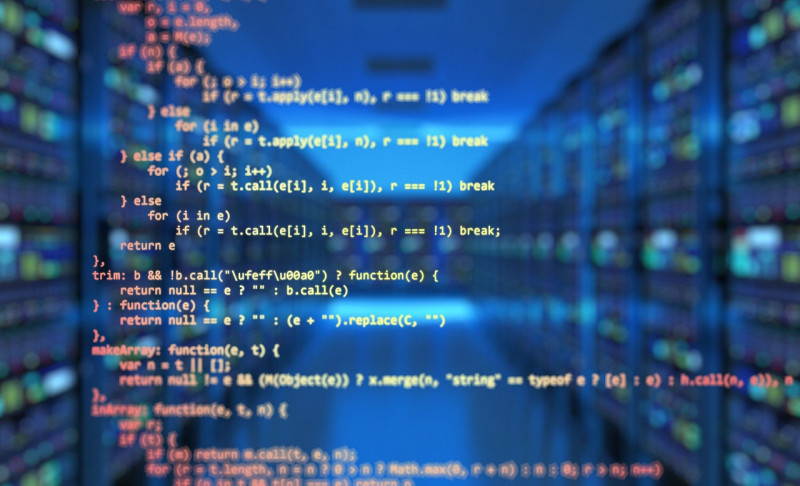
Modelling the Dynamics of Intensive Longitudinal Data (online course) 2024
This online course covers how time series models can be used to model the dynamics of intensive longitudinal data (ILD).
This online course covers how time series models can be used to model the dynamics of intensive longitudinal data (ILD).
This course (and waiting list) is fully booked, so we are unable to accept any new applications.
We focus on ILD from the behavioural sciences, typically collected using questionnaires with ambulatory assessments (AA), the experience sampling method (ESM), ecological momentary assessments (EMA), or daily diaries. We explain the basics of simple and more advanced modelling approaches, the philosophies behind them, and caveats to consider.
The course combines self-study using videos and exercises (in R and/or Mplus), with online Q&A or practical sessions every week. It takes about four hours per week, for a ten-week period. The online meetings will take place on Thursdays between 15-18 CET (with a few exceptions where we will have to host on another day, but within the same time slot)
Technological developments such as smartphones, activity trackers, and other wearables have made it relatively easy to obtain many repeated measurements per person in a relatively short period of time. In response to these measurement innovations, there is a surge of statistical modelling innovations that are designed to handle the unique challenges of such intensive longitudinal data and uncover meaningful properties of these data.
A particular appealing aspect of such data is that the observations are ordered in time, thereby allowing us to study the dynamic relationships between variables over time: how future observations depend on past observations. This can be done with autoregressive time series models for single cases (e.g. individuals or dyads), but also with multilevel extensions for multiple cases. In the latter case, we can additionally study the similarities and differences in the means, variability and dynamics of these different cases. During this course, we discuss these techniques and extensions of these techniques, illustrated with empirical examples from the Social and Behavioural sciences. We focus mainly on techniques for continuous outcome variables.
In the first three weeks of the course, we focus on N=1 time series analysis. Basic topics will be covered, such as the autocorrelation function, (vector) autoregressive models, the ARIMA model, psychological dynamic networks, and stationarity. Building on this basis, we will address extensions of these models that account for measurement error and non-stationary models that account for changing dynamics over time in weeks 4 and 5. In weeks 6 and 7, we discuss continuous time models. In weeks 8 and 9 we consider N>1 approaches, with an emphasis on multilevel extensions of single-subject time series models. In week 10, we will wrap up the course, ending with a consultation panel discussion, where we will look at specific challenges that participants have encountered, and discuss if and how these can be solved. By doing this in a plenary setting, participants will be able to learn from each other’s experiences.
Pre-requisite knowledge
We assume that participants have a solid understanding of multiple linear regression and null hypothesis testing. We also assume participants have a basic knowledge of latent variable modelling (like exploratory or confirmatory factor analysis, or structural equation modelling). Knowledge of multilevel analysis is useful, but not required.
We provide a brief introduction into Bayesian estimation along with this course, to get participants familiar with this approach: Multiple of the discussed techniques, particularly some of the more advanced methods like the multiple subject models, require Bayesian estimation methods (either via DSEM in Mplus or with JAGS via R). Prior experience with Bayesian statistics will hence be helpful to get the most out of this course, but it is not required.
Dr. Noémi Schuurman
Prof. dr. Ellen Hamaker
Dr. Rebecca Kuiper
Dr. Laura Bringmann
Dr. Oisín Ryan
This course is designed for researchers who are interested in gaining more insight into modelling approaches for intensive longitudinal data, with a specific focus on the underlying dynamics (i.e. lagged relationships). While there will be computer labs to obtain some hands-on experience, the emphasis in this course is on obtaining an overview of the diverse challenges associated with these data, and the different philosophies behind the techniques that have been designed to tackle these.
A maximum of 50 participants will be admitted to this course. Please note that the selection for this course will be done on a first-come-first-served basis.
Please note that there are no graded activities included in this course. Therefore, we are not able to provide students with a transcript of grades. You will obtain a certificate upon completion of this course.
The aim of the course is to provide a broad overview of challenges and solutions associated with studying the dynamics in intensive longitudinal data with (extensions of) time series models, in the context of the Social and Behavioural sciences.
Learning goals:
The course combines self-study using videos and exercises (in R and/or Mplus), with either an online Q&A session (one hour) or a practical session (two hours) every week. Participants will spend about four hours per week on the course, for a ten-week period. The online meetings will take place on Thursdays between 15-18 CET (with a few exceptions where we will have to host on another day, but within the same time slot)
Participants can choose to spend more or less time on specific topics in the course.
PhD students from the Faculty of Social and Behavioural Sciences at Utrecht University have the opportunity to attend three Winter/Summer School courses funded by the Graduate School of Social and Behavioural Sciences. Additionally, they may choose to take as many courses as they wish at their own expense from their personal budget.
There are no scholarships available for this course.