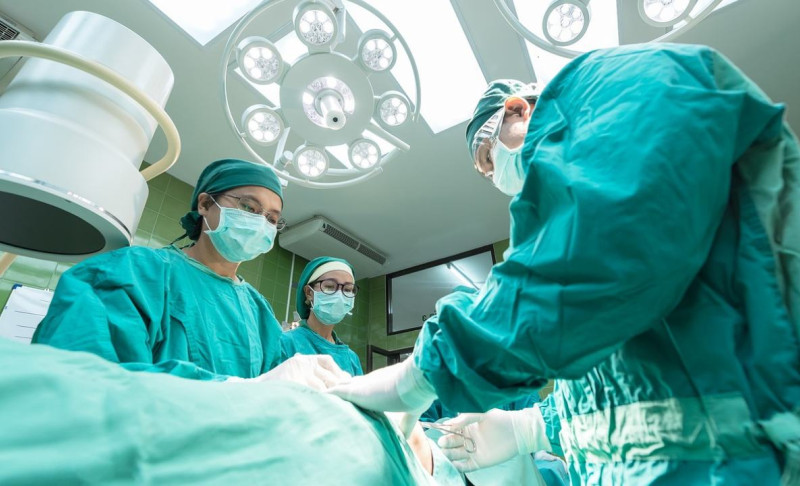
Introduction to Causal Inference and Causal Data Science
The course takes an interdisciplinary approach and is suitable for applied researchers across health, social and behavioural sciences.
The course takes an interdisciplinary approach and is suitable for applied researchers across health, social and behavioural sciences.
During this course, you will learn to view data analysis problems through the lens of causal inference and gain hands-on experience with causal data analysis in R.
How can we learn about causal relations when randomized experiments are unethical, impossible or impractical to conduct? In this one-week in-person course, participants will learn about the latest methods in causal inference with observational data, including: potential outcomes; DAGs and causal graphs; target trial emulation; causal structure learning; quasi-experimental methods; and a variety of methods for handling confounding.
Does exposure to a particular factor cause disease onset? Was the introduction of a new educational policy successful in achieving better student outcomes or not? How should we intervene in a system to achieve some outcome, and what effect can we expect that intervention to have? While data science methods have proven a game changer in performing prediction and classification tasks which tell us what to expect when we passively observe the world, to answer the above causal research questions, researchers must learn to use modern causal inference and causal modeling techniques.
In this five-day on-site summer course, participants will learn about the latest methods for answering causal research questions using non-experimental data, and gain hands-on experience applying these methods to data using R. The course will introduce research to the two main methodological frameworks for causal inference: (1) The potential outcomes framework and (2) Directed Acyclic Graphs (DAGs) together with structural causal models. The latter part of the course will focus on using these basic tools and techniques in more advanced and realistic settings. Participants will learn about the method of target trial emulation to guide the design and analysis of causal inference projects; how causal graphs can be estimated from data using structure learning algorithms; how causal inference principles can guide and interact with prediction modeling techniques from data science; and other advanced topics in causal modeling, such as longitudinal and quasi-experimental settings. By making extensive use of computer labs in R researchers will obtain hands-on practical experience with using these methods.
Dr. Oisín Ryan
Dr. Bas BL Penning de Vries
Dr. Wouter AC van Amsterdam
The course itself takes a broad interdisciplinary approach, and so is suitable for those with a background in health, social and/or behavioural science, or broad training in applied data science. The course is aimed at advanced master level and above, and would be suitable both for data science professionals and academic researchers. As a prerequisite, participants are expected to have a solid basis in statistics and data analysis (regression modelling, general linear model) and working knowledge of R. No prior experience of causal inference techniques is necessary.
When applying, we ask participants to provide a brief motivation letter explaining their background and interest in the course.
Please note that there are no graded activities included in this course. Therefore, we are not able to provide students with a transcript of grades. You will obtain a certificate upon completion of this course
The aim of the course is to introduce participants to the core tools of modern causal modeling methodology. Practical sessions in R will provide participants with hands-on experience applying these methods in practice.
By the end of the week, participants will be able to:
• Define and pose causal research questions
• Represent their substantive knowledge in the form of a causal graph
• Specify a target trial and use this to guide analytic choices
• Investigate whether causal effects are identified in different settings
• Choose which variables they need to adjust for to estimate causal effects
• Estimate causal effects using simple and more advanced confounder adjustment methods in different settings
• Critically evaluate when and what types of causal information can be gleaned from modern data science techniques
• Know how to design and validate prediction models intended for decision support
• Apply causal learning techniques to their data
• Have a working knowledge of different quasi-experimental causal inference techniques
• Apply their knowledge using R
This course has the following fee options, depending on your status:
Participants working in a profit organization: € 1150
Participants working in a non-profit organization: € 930
Participants affiliated to an academic organization (MSc, PhD, researchers): € 820
The housing costs do not include a Utrecht Summer School sleeping bag. This is a separate product on the invoice. If you wish to bring your own bedding, please deselect or remove the sleeping bag from your order.
For this course you are required to upload the following documents when applying: